In a world where information flows at the speed of light, the demands on journalism have never been greater. To navigate this digital age, newsrooms are increasingly turning to the power of AI and machine learning, unveiling a new era of journalism that's as efficient as it is insightful. Imagine a newsroom where AI acts as both editor and fact-checker, tirelessly sifting through vast datasets to bring you the most accurate and timely stories. Welcome to the future of journalism, where technology meets truth, and together, they are shaping the narratives of our world.
Psych!
This is what ChatGPT 3.5 returned when I asked for “an engaging and compelling lede, consisting of 4 sentences, on harnessing AI and machine learning to support efficient production of high-quality journalism.“ First of all, I laughed out loud when I saw “In a world…“ at the top of the lede. If you haven’t seen this movie, stop reading this and go watch it. (Then come back and finish reading please?)
Moreover, blech – this is about as engaging and compelling as an underripe watermelon. And finally, a newsroom where AI acts as both editor and fact-checker – um, thanks but no thanks? Especially on the fact-checking front.
Welcome back to TITLE-ABS-KEY(“science journalism“), a newsletter about science journalism research. In the previous issue, which was admittedly a while ago, I looked at the evolution of journalistic skills and took my chance to make a Taken joke.
This time, as you may have guessed, I’m coming back to AI and journalism, specifically to the issue of trust and whether AI can help meat-based writers increase it.
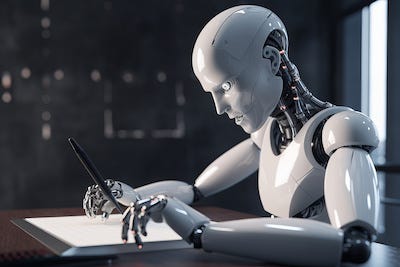
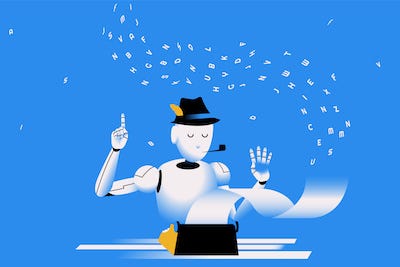
Today’s paper: Opdahl, A. L., Tessem, B., Dang-Nguyen, D. T., Motta, E., Setty, V., Throndsen, E., Tverberg, A., & Trattner, C. (2023). Trustworthy journalism through AI. Data & Knowledge Engineering, 146, 102182. DOI: 10.1016/j.datak.2023.102182.
Why: I mean, the ChatGPT lede sort of confirmed my worst suspicions on this, so I’m even more determined now than I was before when I just hit the right keywords with this paper.
Abstract: Quality journalism has become more important than ever due to the need for quality and trustworthy media outlets that can provide accurate information to the public and help to address and counterbalance the wide and rapid spread of disinformation. At the same time, quality journalism is under pressure due to loss of revenue and competition from alternative information providers. This vision paper discusses how recent advances in Artificial Intelligence (AI), and in Machine Learning (ML) in particular, can be harnessed to support efficient production of high-quality journalism. From a news consumer perspective, the key parameter here concerns the degree of trust that is engendered by quality news production. For this reason, the paper will discuss how AI techniques can be applied to all aspects of news, at all stages of its production cycle, to increase trust.
Oooh, a vision paper. Let me grab my monocle 🧐
In all seriousness, this is a paper on journalism from (mostly) a bunch of computer scientists – and that went so well the last time I did it! This time, there is also a digital marketing expert and a TV journalist in the mix, so let’s see if it works better. I am honestly so interested in the intersection of journalism and AI that I am a very motivated reader (despite the monocle joke.) Plus I think, if we go back to how LLMs work, what the AI-generated lede does show us is that “the future of journalism, where technology meets truth“ is how a lot of human content generators tend to write and think about this. All the more reason to dive into what’s, erm, shaping the narrative of our world.
The last two decades have put pressure on journalists, editors, and newsrooms
. Ah. I’m sorry, was there a hard reset of history in 2000? But okay, okay, they have. The paper goes on to detail this pressure: changing news consumer habits, distrust in authorities, organized disinformation campaigns (kudos for the mention!), news fatigue, and crumbling business models.
Overall it’s a vicious cycle – or even a vicious infinity symbol, ∞, where factors making life worse for journalists (e.g. disinfo campaigns) also make good journalism more important than ever. But! the authors think it’s actually… I don’t know, a shamrock emoji ☘? That is, there’s a third part to this: the same mechanisms that fuel these negative trends at the same time create an opportunity for quality news organisations to thrive.
Interestingly, here’s literally the next sentence in the paper:
This paper presents a vision for how recent advances in Artificial Intelligence (AI) can support trustworthy high-quality journalism at ‘‘every stage of the journalistic value chain.’’
Is it just me or does that subtly imply that recent advances in AI fuel negative trends in media? If so, bonus points for self-awareness. And the value chain in question, as defined by the American Press Institute, is gathering, assessing, creating, and presenting news and information.
The authors go on to mention they aim to contribute to something called AI for Good, which of course doesn’t sound ominous at all, pffft, and cite this paper (It’s a philosophical perspective that is outside the scope of this newsletter, but it’s also from 2018, aka before there were images of the Pope in a puffy jacket, so I’ll have to read it just to see if it stands the test of time.) They provide a one-para literature review, which contains a paper titled AI to Bypass Creativity. Will Robots Replace Journalists? (The Answer Is “Yes”). So, you know, that’s encouraging. Perhaps we’ll get some time off after all1.
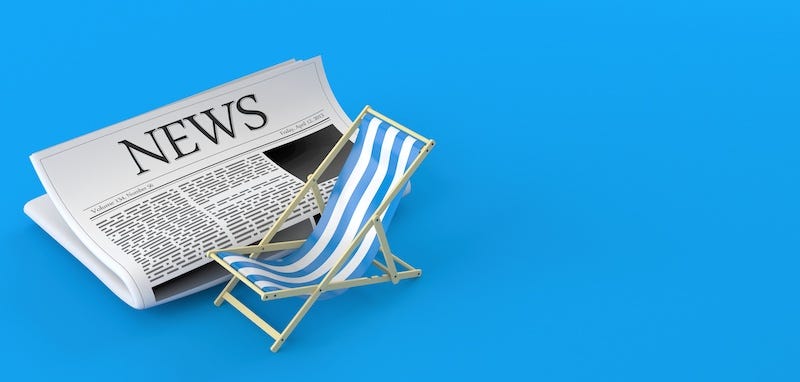
But anyway, the rest of the paper is a discussion of trustworthiness, followed by a look into AI applications in all four parts of the value chain. I’m going to dwell a bit on trustworthiness, in part because it has come up in one of the previous issues.
Honestly, the trustworthiness section of the paper feels very much like a terminology soup that gave me strong “this was produced by AI” vibes. Here’s a list of all related concepts in italics from that section: trust in news media, credibility, fairness, bias, completeness, accuracy, actuality, trustworthiness, ability, benevolence, integrity, legitimacy. The paper does produce a working definition of trustworthiness – here it is:
Trustworthiness of an actor in a domain can be defined as the actor acting responsibly towards people that depend on that actor and on the actor being identifiable and competent in that domain. In the news media domain, this translates into both being and being perceived as fair, handling bias, and reporting in a way that is complete, accurate, and factual.
That’s not too bad, especially in spelling out the crucial distinction between being and being perceived as. But I’m worried that *clears her throat* IN A WORLD where all those characteristics are under vicious and relentless attack, that may not be a very useful definition. If you think you can define and/or explain trustworthiness by using words like fair, I’ve got bad news for you.
A recap of the previous episode, which I’ve linked to above: that study found no difference in perceived credibility and trustworthiness for human and AI authors, either in neutral or evaluative writing. Corn nuts! (yes, that’s yet another polite alternative to swearing from the list I used in that issue.)
So I kind of think that would be good news for this vision paper: if it holds up, then merely adding AI to the toolbox shouldn’t hurt journalists. I’m still not letting it fact-check anything, for sure, but it’s good to know it’s at least (mostly) harmless.
Now, let’s move through problem areas and AI in the value chain. Because there are so many of them, I’m going to use a traffic light system to indicate whether, after giving enough consideration to the authors’ arguments, I would let AI help me, as a journalist, with each activity. I encourage you to go read the cases that interest you the most in full.
Routine harvesting: 🟢. I’d probably have to train any AI-based tool for rather peculiar science journalism needs, but overall I could use some help getting through hundreds of new article alerts per day and two dozen TweetDeck columns. And I’d be prepared for transparency on this front.
Broader harvesting: 🟢. This has to do with tackling unorthodox sources, such as alt news, in addition to your routine monitoring. I guess I would let AI pre-screen content from the bottom half of the science internet? Although that feels cruel to do even to an algorithm.
On-demand gathering: 🟡. In theory, data enrichment for reporting sounds good, but it’s specifically the other scenario in this section – recommending domain experts for a story – that bothers me. Of course, source diversity in science journalism is both crucial and notoriously low for a number of reasons, but it can be very easy to fall into the trap of “unbiased“ AI that will solve all our problems here.
Process automation: 🟡. I admit I feel uneasy about this just because it reads exactly like training the robot journalist to replace you by showing it your work routines. Eh, they probably already know everything anyway.
Data integration: 🟡. So for me this works on a more technical level (I’d let AI deal with tables in a PDF), less so when you need to add opinions and advice to factual content.
Provenance: 🟡. At the end of the day, algorithms can literally be pitted against each other (in a GAN, generative adversarial network), so a provenance investigator AI would be good at this right up until the antagonist AI learns to produce fakes that are convincing enough. And it would not be foolproof against good old-fashioned human mischief.
Fact-checking: 🔴. Yeah, no. Just no. You can’t handle the truth!
Media verification, Deepfakes, Cheapfakes and Cross-modal content verification: 🟡. Same as provenance in general, pretty much. Cheapfakes are part of that human mischief that AI would likely struggle with (does it “know“ you shouldn’t use a 100% real photo of an American soldier in Guam for a May 9th poster?). And I wonder if at least some of the situations that typically require deep cross-modal verification are grave enough (think war crimes investigations) to require a human touch.
Proactive verification: 🟡. I guess this can be used against tampering, but the paper also says this “will require new AI solutions for mining and assessing context,” and we’ll get to context in a bit.
Contribution chains: 🟡. This is close to provenance but more for tracing content back to specific actors. Reading this made me think that coincidences and minds thinking alike (either great in English or dumb in Russian) are still possible, and they often require not just a human mind but a human gut to tackle.
Context: 🔴. This section starts with a rather crude and narrow idea of context (e.g. who owns the original publication), but even there I think AI wouldn’t be good enough now. Broadly, my experience with AI tells me it is incapable of figuring out what proper context even is, and the approaches on the table, such as keyword proximity, recommendation algorithms etc, do not help much.
Source credibility: 🟡. Again, same concerns as with finding and recommending sources.
Retraction: 🟢. Hear me out, I have a very specific need that this can address – I would looooove to have AI alert me if a paper I’ve linked to anywhere in my text corpus got into any trouble and/or has been retracted.
Transparency: 🔴. This is where the paper gets a bit meta, because this is about using AI to explain the outputs of AI elsewhere. I think the very task of explaining is a bit too human for machines at this point, and since AI can’t be responsible or accountable for anything, it also feels odd outsourcing this key task to them.
Real-time assessment: 🟡. This is essentially about getting everyone a good and tireless producer in their ear. Sounds tempting, but also really prone to manipulation.
Robot journalism: 🔴. The authors actually say so themselves: this is not journalism, it’s automatic content creation. I have nothing against it except when it’s blurred into journalism 🤷🏼♀️
Augmented journalism: 🔴. Let’s say I’m convinced by the slippery slope argument from the authors here (more and more augmentation until the augmentee is squeezed out.)
Content units: 🟡. Because I’m curious about structured journalism, I’d carefully dip my toes here I guess.
Trustworthy composition and Suggesting perspectives: 🔴. Both activities are heavily context-dependent, so no.
Live reporting: 🔴. Look, AI is a rookie in journalism, and you don’t let rookies go live unless you absolutely have to.
Iterative journalism: 🔴. According to the paper, this is about developing the story in line with audience insights. I’m hesitant to find out exactly where these stories would iterate, but I might be too cynical.
News discovery: 🟡. First, AI has trouble understanding newsworthiness (because it can’t). Second, it can be a bit of a catch-22 situation: if you can automate news detection, are you going to detect anything truly new?
Narrative generation: 🔴. I like my narratives to come from people, and not when side effects include hallucination, bias, and toxicity.
Contextual presentation: 🔴. See context above.
Trustworthiness reports: 🔴. See fact-checking and provenance concerns above.
Actionability: 🟡. This is an odd and kind of vague one where readers are essentially invited and given tools to fact-check your work – also concerned about human mischief.
News outsiders: 🔴/🟡. This is a combination of AI sensitivity readers (yeah, no, go sit in the corner and then hire a human) and AI accessibility tools (think auto-generated translation or subtitles – again, useful but clearly needs caution.)
Privacy: 🔴. It feels extremely weird to ask AI to detect personal and sensitive information about sources (!) as if its entire MO is not scraping details off everything it touches?…
Monitoring reception: 🔴. I wouldn’t let AI read random comments on my journalism work online, but that’s because I wouldn’t let me read them. I firmly believe in important points and criticism making their way to me via other, less random channels. (Such as your replies to my newsletter!)
Boy, this was a long list. I’ll close by pointing out that at the end of the paper, the computer science crew casually invites journalists onto the slippery slope by saying that “[t]o proliferate in the AI age, journalists and their editors must therefore be encouraged to learn new AI-powered tools and to apply them in their daily work.”
The future science news overlords are coming, I tell ya.
Or not, because the authors conclude that “complete automation of news production will remain unrealistic and unwanted outside of restricted journalistic enclaves, in which the reliance on AI will need to be flagged.“